Best Practices in Quality Assurance: A Complete Guide to Data Excellence
Master proven quality assurance practices that drive real results. Learn battle-tested strategies from industry experts on building robust data governance, automation, and compliance frameworks that actually work.
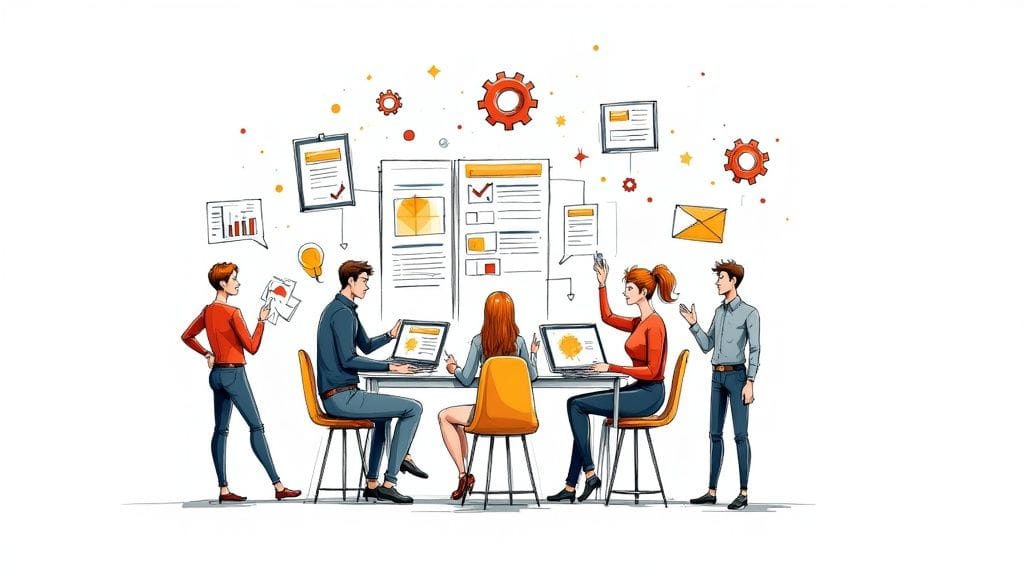
Quality assurance isn't just another business process - it's a mindset that needs to permeate your entire organization. When done right, QA becomes part of your company's DNA, with every team member understanding their role in maintaining data excellence. Let's explore the key building blocks that create this foundation.
Defining Data Ownership and Stewardship
Clear ownership is essential for maintaining high-quality data. Just as a home needs someone responsible for maintaining each room, your data assets need dedicated owners who ensure everything stays in order. For example, your marketing team might manage customer data while finance oversees financial records. This setup creates natural accountability, with specific teams you can turn to when questions arise about particular datasets. Without this clarity, data quality issues often fall through the cracks.
Implementing Effective Data Governance Policies
Data governance policies work hand in hand with ownership roles to keep everything running smoothly. Think of these policies as the house rules - they spell out exactly how data should be handled, from initial entry to storage and access. For instance, you might specify standard date formats or outline steps for double-checking data accuracy. These guidelines should align with industry standards like the UK Code of Practice for Statistics, which emphasizes sensible and transparent QA processes. Getting these policies right helps prevent mistakes while keeping you compliant with relevant regulations.
Creating a Central Data Management Office
A Central Data Management Office (CDMO) serves as your data quality command center. This team doesn't just write rules - they actively help other departments succeed with their data initiatives. They provide training, tools, and guidance to ensure everyone follows best practices. For instance, they might create hands-on workshops about data quality or develop metrics to track improvements over time. The CDMO also helps different teams work together effectively, making sure everyone speaks the same language when it comes to data management. This coordinated approach prevents costly mistakes and helps your organization get maximum value from its data.
Designing Quality Controls That Actually Work
While data governance and policies provide a foundation, success in quality assurance comes down to having controls that work in practice. The key is moving beyond theory to create hands-on systems that actively protect data integrity. Rather than just detecting problems after they occur, well-designed controls prevent issues from happening in the first place. This prevention-focused approach helps maintain smooth operations and builds confidence in the data.
Proactive Monitoring: Catching Issues Before They Escalate
Think of monitoring systems as an early warning radar for your data. Instead of cleaning up messes after they happen, good monitoring spots potential problems as they emerge. For example, you can set up automatic alerts that flag unusual data patterns or entries that don't match expected formats. Finding issues early means you can fix them quickly before they cause bigger problems. This real-time monitoring is essential for keeping data accurate and minimizing disruptions.
Meaningful Metrics: Driving Behavioral Change
Metrics are vital signs that show how well your quality program is working. But tracking random numbers isn't helpful - you need metrics that actually matter. Focus on measurements that reveal the true health of your data and encourage better practices. For instance, if you track data entry errors, you might discover where more training would help. You can also tie quality metrics to performance reviews to make accurate data a priority. Review and update these measurements regularly to make sure they keep driving improvements.
Feedback Loops: The Engine of Continuous Improvement
Quality control works best as a two-way conversation. Set up clear channels for teams to report issues and suggest better ways of doing things. Something as simple as an online form can let employees flag potential errors or recommend workflow improvements. This helps create shared ownership of data quality across the organization. Regular analysis of this feedback often reveals patterns that point to deeper issues, helping you develop more effective controls over time.
Building a Practical Control Framework
Put these quality assurance practices into action with this straightforward framework:
- Risk Assessment: Figure out which data assets matter most and where they're vulnerable
- Control Design: Create specific checks and balances for those key risk areas, from automated rules to manual reviews
- Implementation: Roll out these controls systematically across relevant teams and systems
- Testing and Refinement: Check regularly that controls are working and adjust them based on results and feedback to keep the system effective
When organizations implement these practical quality controls, they create robust systems that both protect and enhance the value of their data. This active approach to managing data quality helps teams make better decisions, run smoothly, and maintain the trust of everyone who relies on their information.
Making Data Audits Work For You
Data audits often get a bad rap as tedious compliance exercises, but they're actually valuable tools for uncovering insights and driving improvements. The key is shifting from a reactive "check-the-box" mindset to actively using audits to strengthen your data practices. When done right, audits help verify that your quality controls work as intended while spotting issues before they become problems.
Establishing Effective Audit Schedules
Your audit timing should match your specific risks and needs. A financial services company handling sensitive customer data might need quarterly reviews, while a marketing agency could do annual audits. Start with yearly audits as a baseline, then adjust based on factors like system changes, new regulations, or past issues. Focus extra attention on high-risk areas - this helps you use resources wisely and catch problems where they matter most.
Identifying Patterns and Insights From Data
Regular audits give you a chance to spot trends that aren't obvious day-to-day. Looking across systems and teams often reveals patterns - like certain fields consistently having errors or specific processes causing bottlenecks. For example, you might notice that sales data entry errors spike at month-end, pointing to potential process or training gaps. These insights help you fix root causes rather than just symptoms.
Turning Audit Findings Into Actionable Improvements
The real value comes from acting on what you learn. Turn audit findings into clear next steps with owners and deadlines. If you find inconsistent data formats across databases, create a standard data dictionary and update procedures. Or if certain teams have higher error rates, provide targeted training. The key is moving from "here's what we found" to "here's what we're going to do about it."
Overcoming Audit Challenges and Building Buy-In
Getting teams on board with audits takes work. Many see them as disruptive or a waste of time. Combat this by showing how audits directly improve data quality and decision-making. Share examples of issues caught and fixed through past audits. Be smart about resources by using automation where possible and focusing on your riskiest areas first. With the right approach and messaging, audits become a natural part of maintaining high-quality data rather than a dreaded chore. Regular audits, combined with steady improvements based on findings, help build stronger data practices across your organization.
Using AI and Automation Effectively in Quality Assurance
Once you've established strong data governance and regular audits, the next step is incorporating AI and automation thoughtfully into your quality assurance processes. The key is taking a practical approach - looking beyond the hype to focus on specific ways these tools can improve your QA work while maintaining appropriate human oversight.
Finding the Right Places for Automation
Automation works best for repetitive, rule-based tasks where it can free up your team to focus on more complex analysis. For instance, automated tools can continuously check data against defined rules and immediately flag any issues, reducing manual effort while improving accuracy. Machine learning systems can analyze large datasets to spot unusual patterns and potential problems before they become serious issues. As these systems process more historical data over time, they get better at recognizing concerning patterns early - letting teams address problems proactively rather than reactively.
How AI Helps With Real Data Validation
Take a typical e-commerce site processing thousands of orders daily. Manually checking every transaction isn't feasible, but AI systems can automatically verify critical data like payment details, shipping information, and product codes in real-time. This immediate validation helps maintain data quality and prevents costly mistakes from slipping through. The AI can also track key metrics over time, alerting teams when something looks off compared to normal patterns. This early warning system helps catch and fix issues quickly before they impact the business.
The Essential Role of Human Oversight
While AI brings major benefits, human judgment remains crucial for quality assurance. Automated systems can only work with what they've been trained on and may struggle with unexpected scenarios or nuanced situations. This is where human expertise makes the difference. For example, while AI can identify suspicious transactions for review, it takes human analysts to investigate the details and make informed decisions about how to handle them. Like an airplane's autopilot, automation handles routine operations well but needs human control for critical moments and unusual circumstances.
A Framework for Adding New Technology
Successfully bringing AI and automation into your QA process requires careful planning. Start by identifying specific pain points where automation could help most. Then evaluate potential solutions based on how well they fit your needs, resources, and existing systems. Consider factors like whether the solution can grow with you and how much training your team will need. Set clear metrics to measure success and regularly check that the automated systems are delivering the expected benefits. With ongoing monitoring and adjustments, you can get the advantages of automation while keeping the human insight that's essential for effective quality assurance.
Navigating the Compliance Maze While Managing Risk
Quality assurance requires careful alignment between compliance requirements and daily operations. Companies that see compliance as part of their core quality strategy, rather than an obstacle, tend to achieve better results in both areas. Let's explore how successful organizations tackle this challenge.
Building Compliance Into Your Processes From the Start
The best time to address compliance is at the beginning, not as an afterthought. For instance, when building a new data entry system, include automatic validation checks that enforce required formats. This prevents invalid data entry and reduces the need for corrections later. Such preventive measures become part of your team's natural workflow, reinforcing good practices without creating extra work.
Developing Risk Management Approaches That Actually Work
Good risk management goes beyond listing potential problems - it requires careful evaluation of both likelihood and impact. Consider a company that handles sensitive customer data. They might identify data breaches as their biggest risk and respond with specific protections like multi-factor authentication, data encryption, and regular security checks. By focusing resources on the most critical risks first, they protect what matters most while staying practical about constraints.
Creating Documentation That Satisfies Auditors Without Overwhelming Your Team
Documentation shouldn't feel like busywork. Think of it like organizing a library - everything needs a clear place and purpose. Using standard templates for common procedures, like data validation steps, ensures consistency while saving time. When auditors arrive, they can quickly find what they need because the information is organized logically. This makes reviews smoother for everyone involved.
Learning From Real-World Successes
Looking at how other companies succeed can provide valuable insights. Take the case of a bank that added automated fraud detection - this improved their regulatory compliance while also reducing fraud losses and building customer trust. Or consider a healthcare provider that created strong data governance rules, with strict access controls and regular audits, to protect patient information under HIPAA requirements. These examples show how compliance can strengthen overall quality when approached thoughtfully. By studying such cases, companies can adapt proven methods to their own needs while avoiding common pitfalls.
Measuring Success and Proving Your Impact
After setting up strong quality assurance processes, you'll need to show they're working and creating real value. Moving beyond seeing QA as just a cost, this section explores concrete ways to track your results, share wins with leadership, and make the case for ongoing QA investment.
Defining Key Performance Indicators (KPIs) for Quality Assurance
Choosing the right KPIs is essential for measuring success. Your metrics should map directly to both your QA program goals and broader business objectives. For example, if you want to reduce data quality issues that customers report, track the monthly count of related support tickets. Or measure what percentage of your data meets quality standards to gauge accuracy. Clear KPIs like these help you monitor progress and prove your quality practices are working.
Tracking and Demonstrating Return on Investment (ROI)
While KPIs tell part of the story, showing ROI is what really matters to the business. Look for ways to connect better data quality to bottom-line benefits. Fewer data entry mistakes mean less wasted time fixing errors. More accurate data leads to smarter business choices. When you can tie QA improvements to actual cost savings and better outcomes, you transform quality assurance from an expense into a strategic asset worth investing in.
Communicating Effectively With Leadership
When sharing results with executives, keep it focused and meaningful. Skip the technical details and highlight key findings that matter for the business. Simple charts and graphs work well to show trends, like declining error rates over time. Match your message to what leadership cares about most - this helps build understanding and support for quality assurance work.
Building a Culture of Continuous Improvement
Keep refining your approach based on what the metrics show. Regular reviews of KPIs, ROI data, and stakeholder feedback reveal where you can do better. Maybe certain teams need more training, or some quality checks could work better. For example, if you spot ongoing data issues in one department, provide targeted help to fix the root cause. Stay flexible and keep improving - this cements quality assurance as crucial to your company's success.
Ready to simplify your development workflow and boost code quality? See how Mergify can help your team merge code smoothly and reduce CI costs. Visit https://mergify.com to learn more and request a demo.